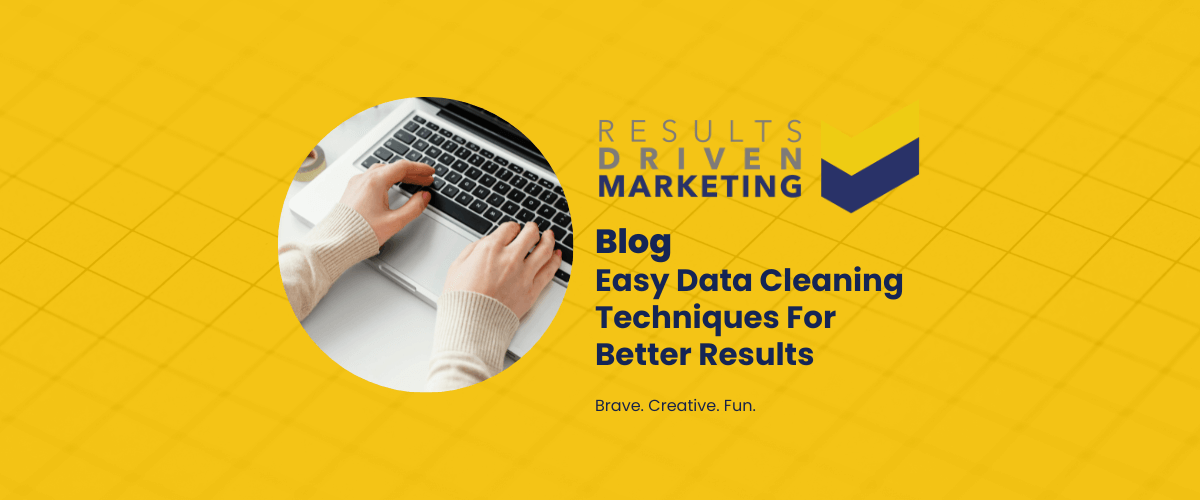
Easy Data Cleaning Techniques For Better Results
The importance of data-cleaning techniques cannot be overstated. As the volume of data continues to grow exponentially, ensuring that this data is accurate, complete, and reliable becomes a critical task for businesses and researchers alike.
Data cleaning techniques are essential for transforming raw data into a refined form that can drive meaningful insights and support decision-making processes.
In this article, we will explore various data cleaning techniques, highlighting their significance, methodologies, and best practices to help you maintain the highest quality data for your analytical needs.
Table of contents:
Why are Data Cleaning Techniques Important?
- Accuracy and Reliability: At the heart of any analysis or decision-making process lies b2b data. Ensuring this data is accurate and reliable is paramount. Data cleaning techniques ensure that the information you’re working with is both trustworthy and actionable.
- Efficiency: Clean data translates to efficient operations. Whether you’re running analytics, machine learning algorithms, or simple database queries, clean data ensures faster processing times and more accurate results.
- Enhanced Decision-making: With clean data at your fingertips, decision-making becomes a more streamlined process. You can trust the insights derived from your data, leading to better and more informed decisions.
Nine Ways to Clean Your Data
In the vast realm of data, the devil, as they say, is in the details. Ensuring that each data point, each column, and each row is in its prime state is not just a task; it’s an art. An art that, when mastered, can lead to unparalleled insights and informed decisions. So, let’s embark on this journey of data cleaning, exploring the top ten ways to ensure your data is as pristine as a freshly cleaned canvas, ready for the masterpiece of analysis.
Spell Checking
- Why it matters: A simple typo can change the entire meaning of a data point. Imagine the difference between ‘sale’ and ‘sail’.
- How to do it: Use data cleaning tools, or even the trusty spell check function in Excel, to spot and rectify any spelling errors. Ensure that industry-specific terms are added to your dictionary to avoid unnecessary corrections.
Finding and Replacing Text
- Why it matters: Sometimes, data might have consistent errors or outdated terms that need updating.
- How to do it: Use the ‘Find and Replace’ function, available in most data cleaning software and Excel, to quickly replace outdated or incorrect terms with their correct or updated counterparts.
Changing the Case of Text
- Why it matters: Consistent capitalisation ensures uniformity and can help in data processing.
- How to do it: Use data cleaning functions in Excel or other tools to change the case of text, be it converting to upper case, lower case, or title case.
Removing Spaces and Nonprinting Characters from Text
- Why it matters: Extra spaces or nonprinting characters can lead to discrepancies in data processing.
- How to do it: Use data cleaning techniques to trim spaces, especially leading or trailing ones. For nonprinting characters, tools like Excel offer functions to spot and remove them.
Fixing Numbers and Number Signs
- Why it matters: Incorrect numbers or signs can skew your analysis.
- How to do it: Validate numbers against a known range or standard. For signs, ensure that they are consistent, especially in financial data where positive and negative values can have significant implications.
Fixing Dates and Times
- Why it matters: Dates and times are crucial for time series analysis, trend spotting, and more.
- How to do it: Ensure a consistent date and time format across your dataset. Handle discrepancies like different time zones or date formats using data cleaning techniques.
Merging and Splitting Columns
- Why it matters: Sometimes, data might be split across multiple columns or merged into a single one, making analysis challenging.
- How to do it: Use tools to split columns based on delimiters or merge multiple columns into one. Ensure that the resulting data is consistent and accurate.
Transforming and Rearranging Columns and Rows
- Why it matters: The arrangement of data can impact readability and processing.
- How to do it: Rearrange columns or rows based on relevance or logical flow. Transform data, if needed, to ensure it’s in the desired format or structure.
Reconciling Table Data by Joining or Matching
- Why it matters: In databases, data might be spread across multiple tables. Reconciling this data is crucial for a holistic analysis.
- How to do it: Use database cleaning processes to join or match tables based on common columns or keys. Ensure that the resulting table is consistent and free of duplicates.
Data cleaning, often seen as a tedious task, is truly the unsung hero of data analysis. It’s the process that ensures your data is not just clean but also meaningful. As we delve deeper into the world of data, remember that every data point, every column, and every row has a story to tell. And with the right data cleaning techniques, that story becomes clearer, more accurate, and infinitely more valuable. Dive in, explore, and let the data guide you.
Components of Quality Data
Quality data is the bedrock of informed decisions. But what makes data ‘quality’? Here are some components:
- Accuracy: Data should be error-free and represent reality.
- Completeness: No missing values or gaps.
- Consistency: Uniformity in format and structure.
- Relevance: Data should be pertinent to the task at hand.
- Timeliness: Current and up-to-date data.
5 Characteristics of Quality Data
- Reliability: Can you trust your data? It should be sourced from reputable sources and be free of errors.
- Validity: Data should be relevant and appropriate for its intended use.
- Uniqueness: No duplicates. Each data point should be distinct and unique.
- Consistency: Uniformity across the dataset, be it in terms of format, structure, or values.
- Accessibility: Data should be easily accessible and retrievable when needed.
What is the difference between data cleaning and data transformation?
At first glance, data cleaning and data transformation might seem like two sides of the same coin. But delve a bit deeper, and the differences become clear. While both are essential in the data preparation process, their goals and techniques differ.
- Data Cleaning: This is all about ensuring the accuracy and quality of data. It involves spotting and rectifying errors, inconsistencies, or missing values. Think of it as grooming your data, ensuring it’s presentable and reliable.
- Data Transformation: This is about changing the format, structure, or values of data to meet specific requirements. It’s like translating your data into a different language, ensuring it’s understandable and usable in a new context.
Advantages and Benefits of Data Cleaning
In the grand tapestry of data analysis, data cleaning might seem like a small thread. But its impact is profound. Here are some advantages:
- Enhanced Decision Making: Clean data offers clear insights, leading to informed decisions.
- Efficiency: Clean data translates to faster processing times and reduced computational costs.
- Trustworthiness: With clean data, you can trust the insights and predictions derived from it.
- Cost Savings: Errors in data can lead to costly mistakes. Data cleaning helps in avoiding such pitfalls.
- Improved ROI: With accurate insights, businesses can optimise strategies, leading to improved returns on investment.
In the end, data cleaning is not just a task; it’s an investment. An investment in accuracy, efficiency, and informed decision-making. So, the next time you’re faced with a dataset, remember the importance of data cleaning and give it the attention it truly deserves. Dive in, refine, and let the data guide you to unparalleled insights.
What are the advantages of data cleaning techniques?
In the bustling world of data, where every byte and bit holds potential value, data cleaning techniques emerge as the unsung heroes. But what makes them so special? Let’s dive in:
- Accuracy Boost: Clean data is accurate data. By eliminating errors and inconsistencies, you ensure that your data truly reflects reality.
- Efficiency Gains: Clean data is like a well-oiled machine. It processes faster, reducing computational costs and time.
- Enhanced Decision Making: With clean data, the insights derived are clearer, leading to more informed decisions.
- Trust Factor: Clean data is trustworthy. Stakeholders can rely on the insights and predictions derived from it.
- Cost Savings: Errors in data can lead to costly mistakes. Data cleaning helps in sidestepping such pitfalls.
What are the key considerations when using data cleaning techniques?
Embarking on the data cleaning journey? Here are some key considerations to keep in mind:
- Scope: Understand the extent of cleaning required. Is it a simple spell-check or a complete overhaul?
- Tools: Choose the right data cleaning tools. Whether it’s Excel for minor tweaks or specialised software for extensive cleaning, the tool matters.
- Validation: Post-cleaning, always validate your data to ensure no errors were introduced.
- Consistency: Ensure that the cleaning techniques used are consistent across the dataset.
- Feedback Loop: Create a mechanism to continuously update and refine your data cleaning techniques based on feedback.
What are the alternatives to data cleaning techniques?
While data cleaning techniques are pivotal, there are alternative approaches to consider:
- Data Masking: Instead of cleaning, mask the data to protect sensitive information.
- Data Augmentation: Enhance the dataset by adding new, synthetic data points.
- Data Anonymisation: Remove personally identifiable information to ensure privacy.
However, it’s essential to note that while these alternatives have their merits, they don’t replace the need for data cleaning.
Data Cleaning Techniques in Machine Learning
Machine learning thrives on data. But not just any data – it requires clean, relevant, and well-structured data to function optimally. Let’s delve into some essential data cleaning techniques used in the realm of machine learning:
Handling Missing Data or Null values.
- Identification: Begin by using data cleaning tools to identify any missing or null values in your dataset.
- Imputation: Depending on the nature of your data, you can use techniques like mean, median, or mode imputation. For instance, data cleaning functions in Excel can be particularly handy for this.
- Deletion: In cases where imputation might not be ideal, consider deleting rows or columns with missing values, especially if they don’t significantly impact your dataset’s integrity.
Handling Duplicate Data
- Spotting Duplicates: Utilise data cleaning software to detect any duplicate entries in your dataset.
- Removal: Once identified, it’s crucial to remove these duplicates to prevent skewed results. Remember, in machine learning, quality trumps quantity.
Dealing with Outliers
- Visualisation: Start by visualising your data. This can help in spotting any apparent outliers.
- Statistical Methods: Techniques like the IQR (Interquartile Range) can be employed to detect and handle outliers.
- Contextual Understanding: Sometimes, what might seem like an outlier could be a genuine data point. It’s essential to understand the context before making decisions.
Erroneous Data
- Validation Checks: Implement validation checks to spot any erroneous data. This could be as simple as spotting a date of birth in the future or a negative value where only positive ones should exist.
- Correction: Once identified, use data cleaning techniques to correct these errors, ensuring your data aligns with the real-world scenario it represents.
Removal of Irrelevant Data
- Relevance Check: Not all data is useful. Conduct a thorough relevance check to identify any data points that might not be pertinent to your analysis or machine learning model.
- Trimming: Post identification, trim your dataset by removing these irrelevant data points. This not only makes your dataset leaner but also more focused.
Data Cleaning Process in Data Mining
Data mining, the art of extracting meaningful patterns from vast datasets, is only as good as the data it operates on. The data cleaning process in data mining is, therefore, a pivotal step. Let’s delve into the steps involved:
- Understanding the Data: Before you even begin the data cleaning process, it’s essential to understand the nature of your data. What does each column represent? What kind of values should each row contain?
- Identifying Anomalies: Using visualisation tools or simple database queries, spot any apparent anomalies. This could be missing values, duplicates, or outliers.
- Data Cleaning Steps:
- Imputation: Fill in missing values using statistical methods or data cleaning functions in Excel.
- Normalisation: Ensure data is on a consistent scale, especially if you’re dealing with varied data types.
- Transformation: Convert data into a format suitable for your data mining algorithms.
- Validation: Post-cleaning, validate your data to ensure no errors were introduced during the cleaning process.
Data Cleaning Techniques in Data Warehouse
A data warehouse, by its very nature, collates data from diverse sources. This amalgamation can often lead to inconsistencies, making data cleaning techniques crucial. Here’s a look at the process:
- Source Identification: Understand where each data point in your warehouse originates from. This can help in tracing back errors to their source.
- Database Cleaning Process:
- De-duplication: Remove any redundant data points that might have been introduced during the data collation process.
- Standardisation: Ensure data from different sources conforms to a standard format.
- Validation: Cross-check data points against their source to ensure accuracy.
- Continuous Monitoring: Given the dynamic nature of data warehouses, it’s essential to continuously monitor and clean your data.
Data Cleaning Tools
The right tools can make the data cleaning process more efficient and accurate. Here’s a look at some popular tools:
- Excel: With its array of data cleaning functions and formulas, Excel remains a favourite for many. Whether it’s spotting duplicates or filling in missing values, Excel’s got you covered.
- OpenRefine: A powerful tool for working with messy data, OpenRefine helps you explore large datasets with ease.
- Trifacta: This tool offers a wide range of features, from structuring semi-structured data to cleaning and validating the data.
- DataWrangler: A tool designed for cleaning and rearranging complex data sets, making them more suitable for analysis.
5 Data Cleaning Project Ideas to Help You Master the Art of Data Cleaning
Looking to get hands-on with data cleaning? Here are five project ideas:
- E-commerce Database Cleaning: Dive into an e-commerce dataset. Spot and rectify missing values, incorrect product categorisations, and inconsistent pricing data.
- Social Media Data Cleaning: Analyse social media data for a brand. Handle missing values, spot and remove bots, and standardise user comments for sentiment analysis.
- Financial Data Cleaning: Work with a dataset from the stock market. Handle missing values, outliers, and ensure data consistency across different stock exchanges.
- Healthcare Data Cleaning: Dive into patient data. Ensure patient records are consistent, handle missing values, and standardise diagnosis codes.
- Real Estate Data Cleaning: Analyse property listings. Handle missing values, standardise property descriptions, and ensure consistent pricing data.
Data cleaning, while often overlooked, forms the backbone of any data-driven decision-making process. Whether you’re diving into data mining, maintaining a data warehouse, or simply looking to understand your data better, mastering data cleaning techniques is crucial. Dive in, get your hands dirty, and remember – clean data is powerful data.
The Further Importance of Data Cleaning Techniques
In the vast ocean of data, ensuring that the information you’re navigating is clean, accurate, and reliable is paramount. But why, you might ask, is data cleaning so pivotal? Well, let’s dive a bit deeper.
Imagine you’re building a house. Would you lay your foundation on unstable ground? Similarly, in the realm of data-driven decision-making, the foundation is your data. If it’s riddled with errors, inconsistencies, or irrelevant information, the insights derived can be misleading, if not entirely incorrect. Data cleaning, therefore, is not just a process; it’s a necessity. It ensures that your data is in its best shape, ready to offer valuable insights and drive informed decisions.
Let’s delve into some specific techniques and steps that play a crucial role in the data cleaning process:
Standardise capitalisation
- Why it matters: Inconsistent capitalisation can lead to discrepancies. For instance, ‘apple’, ‘Apple’, and ‘APPLE’ might be treated as different entities in a database.
- How to do it: Use data cleaning tools or functions in Excel to ensure consistent capitalisation across your dataset. Whether you choose title case, upper case, or lower case, consistency is key.
Convert data type
- Why it matters: Different data types can lead to processing errors. Imagine trying to add a text string to a number; it just doesn’t work.
- How to do it: Identify the desired data type for each column in your dataset. Use data cleaning software or Excel functions to convert data into the appropriate type, be it text, number, date, or any other.
Clear formatting
- Why it matters: Excessive or inconsistent formatting can clutter your dataset, making it harder to read and process.
- How to do it: Strip away any unnecessary formatting. This includes removing bold, italics, or underlined text, ensuring consistent font sizes and styles, and getting rid of any extraneous colors or highlights.
Fix errors
- Why it matters: Errors, be they typos, incorrect values, or misplaced data points, can skew your analysis and lead to incorrect insights.
- How to do it: Use data cleaning techniques to spot and rectify errors. This could involve manual checks, validation rules, or even automated data cleaning processes.
Language translation
- Why it matters: In our globalised world, data often comes from multiple regions, each with its own language. Ensuring that your data speaks a consistent language is crucial for accurate analysis.
- How to do it: Use language translation tools or services to convert data into your desired language. Ensure that translations are accurate and contextually relevant.
In essence, data cleaning is not just about tidying up; it’s about ensuring that your data is a true reflection of reality. It’s about laying a strong foundation for your analysis, ensuring that the insights you derive are both accurate and actionable. So, the next time you’re diving into a dataset, remember the importance of data cleaning and give it the attention it truly deserves.
FAQ
Why are Data Cleaning Techniques Important in Machine Learning?
Machine Learning thrives on data. Clean data ensures that the algorithms learn from accurate, reliable data, leading to more accurate predictions and insights.
How does Data Cleaning Process differ in Data Mining and Data Warehouse?
In Data Mining, the focus is on extracting patterns and insights, so the cleaning emphasises accuracy and relevance. In a Data Warehouse, where data from diverse sources is collated, the emphasis is on consistency and standardisation.
How to ensure the best results with Data Cleaning Techniques?
Regularly review and update your data cleaning techniques, validate post-cleaning, and ensure consistency in the cleaning process.
Why is uniform language important in data cleaning?
Uniform language ensures that the data is consistent, making it easier to process and analyze.
How to validate the data after cleaning?
Use validation rules, cross-check with original sources, and employ data validation tools.
Why is structural consistency crucial in data cleaning?
Structural consistency ensures that the data is in a format that’s easy to process and analyze, ensuring efficient data processing.
How does semantic analysis aid in data cleaning?
Semantic analysis understands the meaning behind the data, helping in identifying inconsistencies or errors that might not be apparent at first glance.
Conclusion
In the digital age, the integrity and accuracy of data have become paramount for companies aiming to make informed decisions. Data cleaning is not just a one-time task but an ongoing commitment to ensure the reliability of databases. To summarise, here are the key points companies need to remember, when they buy b2b data:
- Understanding Data Quality: Recognise the importance of high-quality data for business operations and decision-making. Poor data can lead to misguided strategies and financial losses.
- Regular Audits: Periodically review and audit your data to identify inconsistencies, duplicates, and errors. This proactive approach can prevent larger issues down the line.
- Employ Data Cleaning Techniques: Utilise advanced data cleaning techniques to rectify inaccuracies, fill in missing values, and remove redundant information. These techniques are essential tools in the arsenal of every data-driven organisation.
- Automation: Consider automating the data cleaning process where possible. Automation can ensure consistency, save time, and reduce the possibility of human error.
- Training and Awareness: Ensure that team members are well-trained in data management practices. A well-informed team can prevent many common data errors at the source.
- Backup and Recovery: Always maintain backups of original data before initiating any cleaning process. This ensures that you have a fallback option in case of any unintended data loss.
- Stay Updated: The world of data management is ever-evolving. Stay updated with the latest data cleaning techniques and tools to ensure your databases remain in pristine condition.
In conclusion, as businesses increasingly rely on data-driven insights, the emphasis on data cleaning techniques cannot be overstated. A well-maintained database is not just an asset but a cornerstone for the success and growth of modern enterprises.
Who are we?
Dedicated to lead generation, Results Driven Marketing provides myriad services SMEs can trust to deliver results.
Our marketing lists are guaranteed accurate to industry high standards, and GDPR compliant and our experience team means that if you are looking to buy data, they make them totally bespoke and highly relevant whether you are looking for email lists, direct mailing lists or telemarketing lists.
Our email marketing software is highly rated. Responder provides the automation tools you need to put your marketing on autopilot.
We also supply email marketing solutions with our email marketing platform.
Call us today on 0191 406 6399 to discuss your specific needs.
Results Driven Marketing
info@rdmarketing.co.uk
0191 406 6399
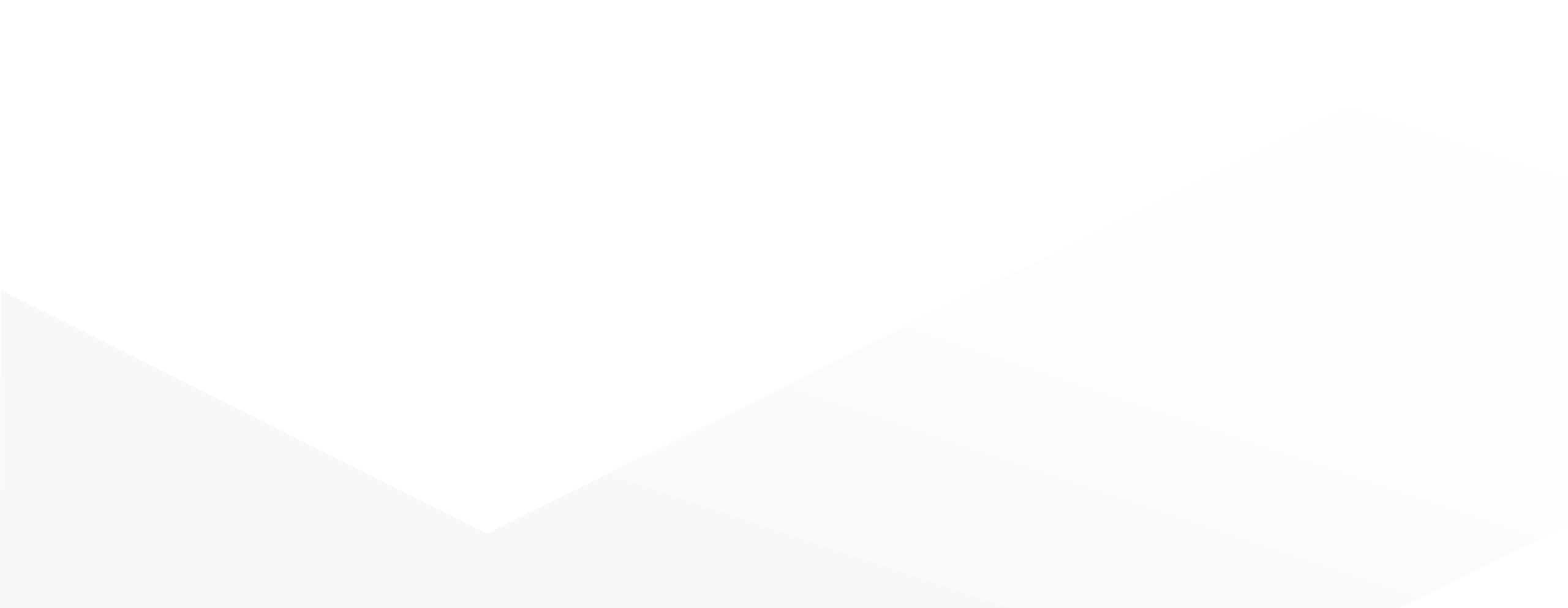